I. Introduction
Cardiovascular and cerebrovascular diseases, along with cancer, are the three major causes of deaths. The mortality rate of diseases of the circulatory system is 117.2 per 10,000. Among diseases of the circulatory system, the mortality rate per 10,000 is in the following order: cerebrovascular disease (59.6), cardiovascular disease (43.7), and hypertensive disease (11.0) [
1]. Moreover, hypertension has the highest prevalence among diseases of the circulatory system. However, over one-half of patients with hypertension are not aware of their disease, and even if they are diagnosed with hypertension, they are not compliant with the recommended management. Indeed, after being diagnosed with hypertension, approximately 20% of patients with hypertension continue with the recommended treatment as prescribed, and over 65% of patients discontinue treatment against medical advice [
2-
4]. Hypertension alone is not important, unlike comorbidities, such as stroke, myocardial infarction, congestive heart failure, and peripheral vascular disease. Of greatest importance, hypertension contributes to the occurrence of cerebrovascular disease (35%) and ischemic heart disease (21%) [
5].
Various conventional studies have shown that hypertension is related to other diseases, such as cerebrovascular and cardiovascular diseases. However, studies demonstrating an association among co-morbidities of hypertension have not been proposed. Therefore, in this study, we determined the relationship among co-morbidities of hypertension based on association rule mining (ARM). ARM is a powerful method to analyze the association among tree and more 3 co-morbidities for the following the reasons: 1) ARM can manage the relationship of several items, and 2) the confidence value can be used in arithmetic operations [
6].
II. Methods
1. Subject of Investigation
In this study, the data of inpatients over 18 years of age with essential hypertension at A hospital in D city was used. The period of data collection was from May 2005 to December 2007 using electronic medical records.
2. The Process of Study and Data Collection
The process based on ARM to analyze patients diagnosed with essential hypertension is shown in
Figure 1. We collected diagnostic data of patients with essential hypertension which were classified into I10 according to International Classification of Disease (ICD) and Korea Classification of Disease (KCD) from the data warehouse (D/W). The personal information, such as name, resident registration number, and telephone number were removed from the data.
3. Constructing Data Mart for Patients with Essential Hypertension
A total of 5,022 patients were diagnosed with essential hypertension and the total diagnostic data numbered 53,994. Moreover, high support for the disease occurred if a patient was diagnosed with the same disease several times. Therefore, we have removed duplicated data by comparing the registration number and diagnosis code. Diagnoses related to external factors, such as injury, poisoning, certain other consequences of external causes (SOO-T98), external causes of morbidity and mortality (V01-Y98), factors influencing health status and contact with health services (Z00-Z99), and codes for special purposes (U00-U99) have been removed from the data mart. Data mart with 26,823 cases was constructed and used for correlation analysis.
4. Analysis Method
The statistical analysis program, SPSS Clementine 12.0 (SPSS Inc., Chicago, IL, USA), was used. Frequency analysis was performed on gender, age, and other diseases of the patients with hypertension. Moreover, Apriori modeling and web node were performed to analyze the strengths of associations among hypertension and other diseases.
Web node is a visualization tool to represent the relationship between items, and Apriori modeling is a modeling method of ARM that makes it possible to apply binominal or multi-nominal data types. ARM is used to analyze the tendency of how often item A and item B occur together. Then the support is defined as the percentage of transactions that contains diagnosis case 1 (Dx1) and diagnosis case 2 (Dx2), and may be regarded as
P (Dx1∪Dx2) which is direction-independent. The confidence is defined as the ratio of the support of the item set (Dx1∪Dx2) to the support of the item set, Dx1, which roughly corresponds to the conditional probability,
P (Dx1|Dx2), and is direction-dependent. In terms of epidemiology, the support resembles the prevalence rate of Dx1 and Dx2 within a certain period of time. The confidence, ratio of the co-occurrence rate of Dx1 and Dx2 over the prevalence of Dx1, resembles the co-morbidity of Dx2 with Dx1 within the same period of time, but is direction-dependent. As a result of the Apriori modeling, association rules are evaluated on the values of support and confidence [
6-
9]:
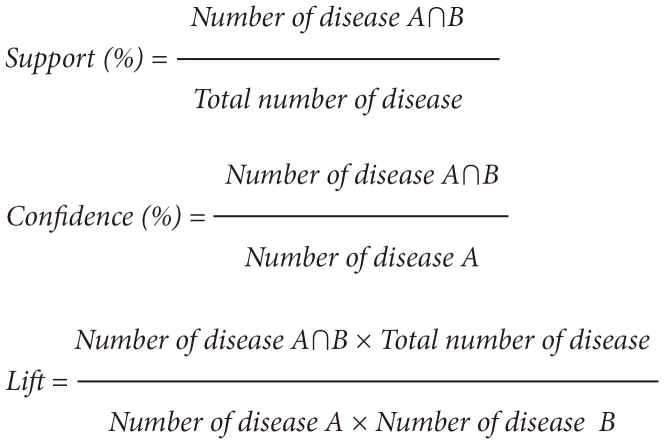
III. Results
1. Patient Gender and Age Distribution
The data consisted of 2,508 males (49.94%) and 2,514 females (50.06%) for a total 5,022 patients. Moreover, in the age distribution, the patients over 70 years of age were the most frequent (1,882; 37.48%), and the patients between over 18 years and less 29 years of age were the least frequent (35; 0.70%), as shown in
Figure 2. The mean and standard deviation was 65 ± 11 years of age.
2. Distribution of Other Diseases in Patients with Essential Hypertension
The frequency of other diseases in patients with essential hypertension is shown in
Table 1. Non-insulin-dependent diabetes mellitus (E11) was the most frequent disease (1,765 patients), and cerebral infarction (I63), angina pectoris (I20), and chronic renal failure (N18) showed a high frequency in that order. In the case of distribution of diseases according to gender, non-insulin-dependent diabetes mellitus was the most frequent disease, and cerebral infarction and angina pectoris showed a high frequency as well. In case of males, acute myocardial infarction (I21) and gastric ulcer (K25) had a statistically significant difference (
p < 0.05), although cerebral infarction (I63), angina pectoris (I20), chronic renal failure (N18), acute myocardial infarction (I21), gastric ulcer (K25), and prostatic hyperplasia (N40) had a higher frequency than females. In the case of females, gastritis and duodenitis (K29), heart failure (I50), and osteoporosis without pathologic fractures (M81) had a statistically significant difference (
p < 0.05); non-insulin-dependent diabetes mellitus (E11), gastritis and duodenitis (K29), disorders of lipoprotein metabolism and other lipidaemias (E78), hemiplegia (G81), heart failure (I50), and osteoporosis without pathologic fractures (M81) showed a higher frequency than males.
3. Result Visualization by Web Node
Figure 3 shows the results of the relationship among essential hypertension and high frequency diseases listed in
Table 1 using web node. Co-morbid diseases were linked with each other. From the results shown in
Figure 3, essential hypertension was linked with non-insulin-dependent diabetes mellitus and cerebral infarction, and non-insulin-dependent diabetes mellitus was linked with cerebral infarction. Therefore, it was shown that non-insulin-dependent diabetes mellitus and cerebral infarction have a relationship with essential hypertension. Other diseases, such as disorders of lipoprotein metabolism and other lipidaemias (E78) and acute myocardial infarction (I21), did not have a relationship with essential hypertension.
4. Results of ARM Using the Apriori Modeling
Based on the results of the Apriori modeling, the association rules among essential hypertension and specific diseases are shown in
Table 2. We extracted 8 association rules and the used threshold values were as follows: support, ≥5%; and confidence, ≥15%. The rule with the highest support and confidence was 'non-insulin-dependent diabetes mellitus to essential hypertension', which had confidence and support values of 100% and 35.15%, respectively. The second rule was 'cerebral infarction to essential hypertension', which had confidence and support values of 100% and 21.19%, respectively. The third rule was 'essential hypertension and cerebral infarction to non-insulin-dependent diabetes mellitus', which had confidence and support values of 37.31% and 7.91%, respectively. The rule for 'essential hypertension and non-insulin-dependent diabetes mellitus to cerebral infarction' had confidence and support values of 22.49% and 7.91%, respectively. The other rules for 'essential hypertension and non-insulin-dependent diabetes mellitus to angina pectoris' and 'essential hypertension and non-insulin-dependent diabetes mellitus to chronic renal failure' had a confidence less than 20%.
IV. Discussion
This study aimed to analyze the association among essential hypertension and other diseases using the Apriori modeling, which is a popular and powerful method in data mining [
10]. In this study, we used 53,994 diagnoses data extracted from the D/W accumulated based on electronic medical records. Therefore, using the D/W was possible to analyze massive data, which was different from an epidemiologic study by reviewing paper-based medical records or a prospective study [
11]. Moreover, this study was meaningful to analyze the association among essential hypertension and various comorbid diseases.
Hypertension is known as a risk factor for diabetes mellitus, cardiovascular disease, and cerebrovascular disease. In this study, the results based on web node showed that essential hypertension, non-insulin-dependent diabetes mellitus, and cerebral infarction have a relationship with each other. Based on the results of the Apriori modeling, the association rule for 'essential hypertension to non-insulin-dependent diabetes mellitus' had the highest confidence and support. Thus, essential hypertension and non-insulin-dependent diabetes mellitus were associated with one another. Lee and Park [
12] stated that 39% of first-diagnosed diabetes mellitus patients had co-morbid hypertension. The patients with hypertension had a 2.5-fold higher prevalence than people with normal blood pressure, and hypertension occurred 3-fold higher in patients with diabetes mellitus. Therefore, the patients with either hypertension or diabetes mellitus need to care for both blood pressure and blood glucose together because the comorbidity of hypertension and diabetes mellitus could be the basis for the increased clinical attack rate of cardiovascular disease and cerebral infarction, myocardial infarction, heart failure, and renal failure [
13]. In another study [
12] it was reported that >80% of patients with diabetic microangiopathy or diabetic nephropathy had hypertension as a co-morbidity, and patients with hypertension had a 2-fold higher attack rate for coronary artery disease and a 2-6-fold higher attack rate for cerebrovascular disease than non-diabetics of the same age group [
12]. In this study, we investigated the relationship between essential hypertension, non-insulin-dependent diabetes, and other diseases based on ARM. Based on the results, we showed that essential hypertension and non-insulin-dependent diabetes influenced co-morbid cerebral infarction, angina pectoris, and chronic renal failure.
We have applied ARM to a large electron medical record data base of patients with hypertension to analyze the association with co-morbid diseases. However, the data that we used in this study were the inpatients' clinical records of the one hospital located in D city. Moreover, it was difficult to analyze sequential patterns because patients were diagnosed with several diseases at the same time in some cases. Therefore, studies based on data collected from various hospitals to find out general and sequential rules will be the subject of further studies.
Acknowledgements
This work was supported by the grant No. RTI04-01-01 from the Regional Technology Innovation Program of the Ministry of Knowledge Economy (MKE).
References
1. National Statistical Office of Korea. Annual report on the cause of the death statistics 2008. 2008. Seoul: National Statistical Office of Korea; p. 1-255.
2. Lee SW, Kam S, Chun BY, Yeh MH, Kang YS, Kim KY, Lee YS, Park KS, Son JH, Oh HS, An MY, Lim PD. Therapeutic compliance and its related factors of patients with hypertension in rural area. Korean J Prev Med 2000;33:215-225.
3. Yoon SJ, Ha BM, Kim CY. Measuring the burden of hypertension using DALY in Korea. Korean J Health Policy Adm 2001;11:89-101.
4. Hwang EH. The study of risk factors of the disease worse due to hypertension and management aspect [dissertation]. 2003. Chuncheon: Kangwon National Univ.
5. Jee SH, Suh I, Kim IS, Appel LJ. Smoking and atherosclerotic cardiovascular disease in men with low levels of serum cholesterol: the Korea Medical Insurance Corporation Study. JAMA 1999;282:2149-2155. PMID:
10591337.
6. Tai YM, Chiu HW. Comorbidity study of ADHD: applying association rule mining (ARM) to National Health Insurance Database of Taiwan. Int J Med Inform 2009;78:e75-e83. PMID:
19853501.
7. Kang HC, Han ST, Choi JH, Kim ES, Kim MK, Lee SK. Data mining with SAS Enterprise Miner 4.0: methodology and application. 2002. 3rd ed. Seoul: Jayuacademi; p. 155-174.
8. Heo MH, Lee YG. Data mining modeling and case. 2008. 2nd ed. Seoul: Hannarae; p. 171-250.
9. Bae HS, Cho DH, Suk KH, Kim BS, Lee JY, Noh SW, Lee SC, Chon YH. Data mining using SAS Enterprise Miner. 2008. 2nd ed. Seoul: Kyowoosa; p. 80-86.
10. Chobanian AV, Bakris GL, Black HR, Cushman WC, Green LA, Izzo JL Jr, Jones DW, Materson BJ, Oparil S, Wright JT, Roceella EJ. Seventh report of the Joint National Committee on Prevention, Detection, Evaluation, and Treatment of High Blood Pressure. Hypertension 2003;42:1206-1252. PMID:
14656957.
11. Choi JW, Lee YH, Kim KJ, Kim JS, Park JS, Song JH, Lee EJ, Kim SG, Kim JD, Kim SG. The analysis of clinical information by building the clinical data warehouse. J Korean Soc Med Inform 2001;7:1-11.
12. Lee SM, Park RW. Basic concepts and principles of data mining in clinical practice. J Korean Soc Med Inform 2009;15:175-189.
13. Lee HJ. Hypertension: the role of hypertension in diabetic patients, the cause and etiology. Clin Diabetes 2004;5:215-219.